Avoid These Five Behaviors That Make You Look Like A Data Novice
And be a trustworthy, likable data partner
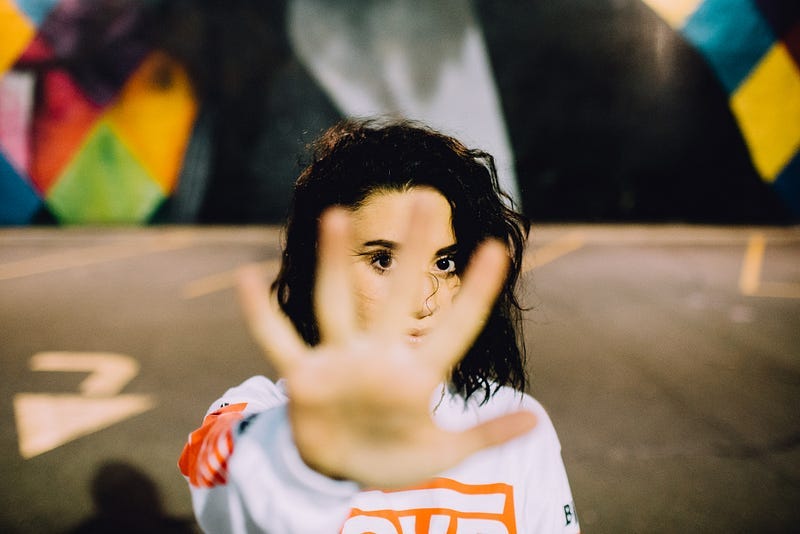
Opinion
And be a trustworthy, likable data partner
With more and more data being collected every day and virtually every company priding itself on making data-driven decisions, data is at everyone’s fingertips. Data Science is becoming a hotter and hotter field by the day. You are here reading this probably because you are enthusiastic about data and want to be able to develop expertise in the field. With all the boot camps and online classes nowadays, everyone can feel like a data expert in months or even weeks; but being a truly helpful, likable, and credible “data partner” to co-workers and other stakeholders takes more than just familiarity with SQL and Python and basic stats knowledge.
There are noticeable differences between people who are new to the data world and those who truly understand how to handle data and be helpful data partners. I have observed people who demonstrate behaviors that amount to no less than waving your arms in the air and screaming “I’m new to this, I have no idea what I’m doing…”. And I have done most of these things myself when I first started as a data scientist. These behaviors can diminish your credibility as a data partner quickly and make people question your understanding of the subject matter. So hopefully I can provide some advice for what NOT to do and what to do instead so you don’t become THAT data person.
#1 Over-interpreting results and trying to make up stories out of nothingness.
“We have deduced that there’s a positive correlation between X and Y… based on 30 data points, and we believe it’s due to…” I die a little whenever I hear people make statements like the one above. When it comes to trend analysis and generating insights, the sample size is always the number one thing to consider. Unless it’s a focus group with people that are representative of your customer base (I even have doubts about survey results from focus groups, but that’s another topic), 30 data points usually won’t give you any robust insights.
Is there anything more embarrassing than deducing “trends” from extremely small datasets? Yes, coming up with theories for why these “trends” are happening. I have seen people come up with all sorts of wild theories to explain why the results from tiny datasets are “counter-intuitive”; they lose their credibility along with most of the audience in the process when the real explanation is simple… it’s simply noise.
Try this instead: Instead of jumping into trend analyses when the sample is small, focus on setting up structures to collect more data with better quality going forward to enable those analyses in the future. If you REALLY want to have some insights coming out of the small sample, caveat your finding with the lack of sample quantity and add a confidence interval to metrics that you report.
#2 Not quality checking (QC) the data/query before using it.
There are no perfect datasets out there; anyone who tells you otherwise is either lying or doesn’t know better. So as a data expert, you should know better than trusting data quality at face value. EVERY SINGLE PIECE of data you query and analyze needs to be quality checked — make sure tables are ACTUALLY deduped as they should be, check that timestamps are in the timezone you THINK they are, and so on. Not performing QC on the data before using it can cause unintended results and misleading insights and make people doubt your ability to deal with complicated data.
Try this instead: Develop a QC framework (i.e. a list of tests you perform) and go through it every time you work with a new dataset. For example, check for (unexpected) duplicates; if you expect the data set in question to have one row per customer order, write a quick query to group by order id and count the number of rows — you will be surprised how many “order-level” tables have 1,000 records for some order ids. Always, always, always sanity-check your work; and double sanity-check with your stakeholders and subject matter experts.
#3. Over-engineering things.
I still remember the excitement after I learned about fancy models like the Random Forest or XGBoost; when you have a hammer, especially a shiny cool one, everything looks like a nail. But in reality, unless you are an ML engineer, you rarely need 10-layer neural networks in your day-to-day data work. Using fancy ML models when a simple linear regression suffices is not only inefficient but also counter-productive. As I mentioned in my article about data science lessons I learned from working at McKinsey, making a business impact is the number one goal when it comes to working as a data scientist in the industry, not showing off how much ML knowledge you have.
Over-engineering models and analyses is a surefire way to make yourself the unpopular and ineffective data partner people want to avoid working with.
Try this instead: Start simple, and only apply more complex methods if it’s truly necessary. Make very conscious decisions about the methodology you use in analyses, and apply the 80/20 rule to avoid unnecessary efforts that only bring marginal benefits, if at all.
#4. Buzz word dropping.
This one is very common among people who just entered the data world. Similar to having the tendency to over-engineer things out of excitement about the new modeling skills, a lot of new data practitioners like to use all the new concepts and new words they learned whenever possible. When communicating, we tend to make up for our lack of understanding of things with complexity — the more buzz words a person uses when talking about ML and analytics, the less analytics he/she usually knows. A seasoned data practitioner should be able to explain the methodology and analytical details in plain English; if someone’s explanation of data work is as hard to understand as reading a Wikipedia page, it’s probably because they just read about it on Wikipedia too.
Try this instead: When learning about a new analytical concept, really try to understand it to the point that you can easily explain it to your friends who are not data scientists… in plain English. This level of understanding will also help you decide when to apply the fancy but complicated approach and when to use the good old-fashioned linear regression.
#5. Ignoring stakeholders’ needs when creating data products.
Occasionally I meet new data practitioners who don’t only suffer from symptoms 3 and 4 above but carry their overzealousness so far as to create data pet projects at work that nobody appreciates but themselves. Don’t get me wrong, I think all enthusiasm in data should be encouraged, and pet projects are fun and helpful in developing skills…just not at your day job, where a business is counting on you to use data products to drive impact.
Data products (e.g. dashboards) are just like any other product, the number one design rule for them should be user-centricity. They should be born out of needs…not just passion.
Try this instead: Talk to your stakeholders before building any data product. Understand the business’ needs at the current stage: If it’s a startup, I bet your stakeholder won’t care too much about the format and color of the data visualizations you build but wants to instead focus on the accuracy of the data behind the visualizations and insights from them. Similarly, truly understand the audience and use case; for example, you would spend more time on a polished and simple user interface if the data product is intended to be used regularly by non-technical audiences.
Develop your pet projects on the side, maybe it will come in handy someday; just don’t let them get in the way of you being an effective and likable data partner.
Key takeaways
Don’t over-complicate or over-engineer things; it will NOT make you look smart, it will make you look like you don’t know what’s the most effective way of doing things.
Make sure to QC your data and sanity-check your insights and always caveat findings when data quality or the sample size is a concern.
Have your stakeholders in mind when creating data products.